Generative Adversarial Network
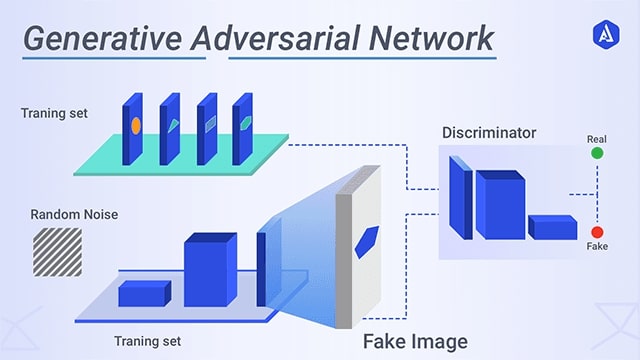
A generative adversarial network (GAN) is a class of machine learning frameworks designed by Ian Goodfellow and his colleagues in June 2014. Two neural networks contest with each other in a game (in the form of a zero-sum game, where one agent's gain is another agent's loss).....Coming soon with new material.
$\frac{1}{2}$